The PCR-based blood test
Using computational and machine learning, including analysis and integration of tumor tissue and circulating peripheral blood transcripts, we identified a panel of 51 marker genes selectively associated with GEP-NENs.
GEP-NEN gene co-expression network
Visualization of the GEP-NEN gene co-expression network and heatmap visualizing enrichment for over-represented Gene Ontology (GO) Biological Process (BP) terms.
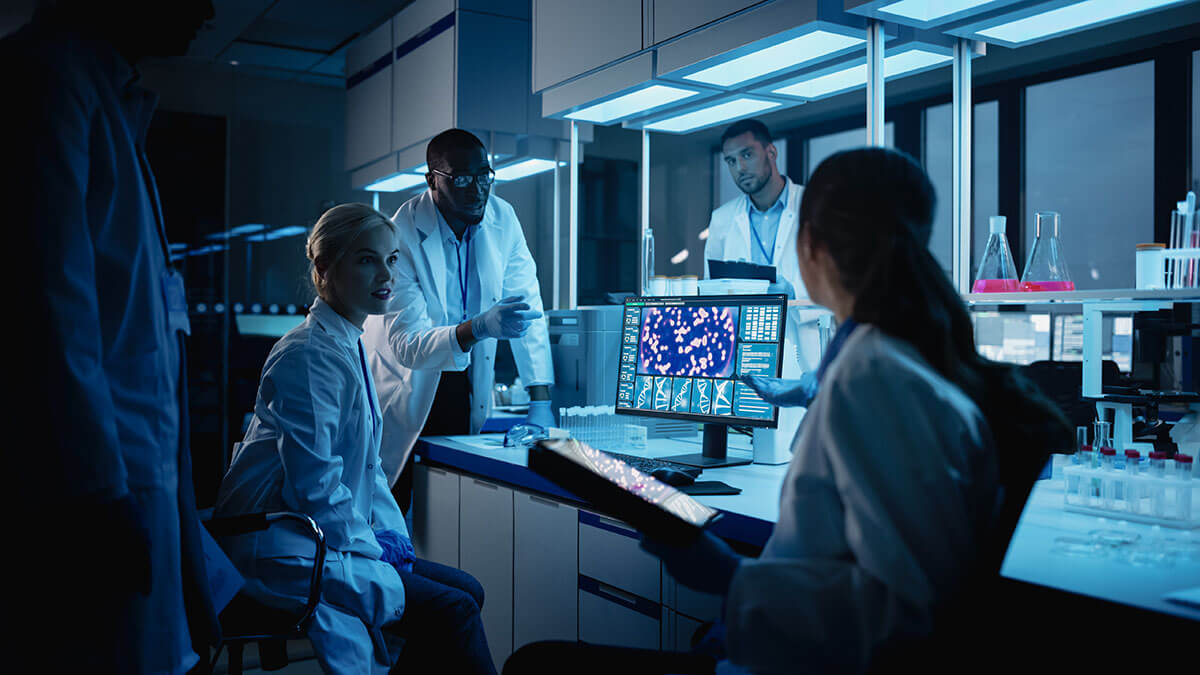
PCR tests
Using computational and machine learning, including analysis and integration of tumor tissue and circulating peripheral blood transcripts, we identified a panel of 51 marker genes selectively associated with GEP-NENs. This can differentiate between GEP-NENs and controls and has a high PPV (>90%).
Computational pipeline used to derive a set of 51 markers that identify GEP-NEN disease.
Step 1: Gene co-expression networks inferred from GEP-NEN-A and GEP-NEN-B datasets are intersected, producing the GEP-NEN network.
Step 2: Co-expression networks from neoplastic and normal tissue microarray datasets are combined to produce the normal and neoplastic networks.
Step 3: Links present in normal and neoplastic networks are subtracted from the GEP-NEN network.
Step 4: Concordantly regulated genes in GEP-NEN-A and GEP-NEN-B networks are retained; other genes are eliminated from the GEP-NEN network, producing the Consensus GEP-NEN network.
Step 5: Upregulated genes in both the GEP-NEN-A and GEP-NEN-B dataset are mapped to the Consensus GEP-NEN network.
Step 6: Topological filtering, expression profiling, and literature-curation of putative tissue-based markers, yielding 21 putative genes further examined by RT-PCR.
Step 7: Identification of mutually up-regulated genes in GEP-NEN blood transcriptome and GEP-NEN-A GEP-NEN-B datasets, yielding 32 putative genes further examined by RT-PCR.
Step 8: Literature-curation and cancer mutation database search, yielding a panel of 22 putative marker genes for further RT-PCR analysis.
The PCR-based blood test exhibited correct call rates of 91-97% with sensitivities and specificities of 85-98% and 93-97% for the identification of GEP-NENs.
A PCR-based blood test is significantly more sensitive than other blood measurements and can detect the majority (~95%) of patients with disease irrespective of the location, extent, grade or metastasis
Test utility: a “rule-out” diagnostic test (to confirm absence of a GEP-NEN or residual disease) and as a prognostic test to measure therapeutic responses to individual treatments.
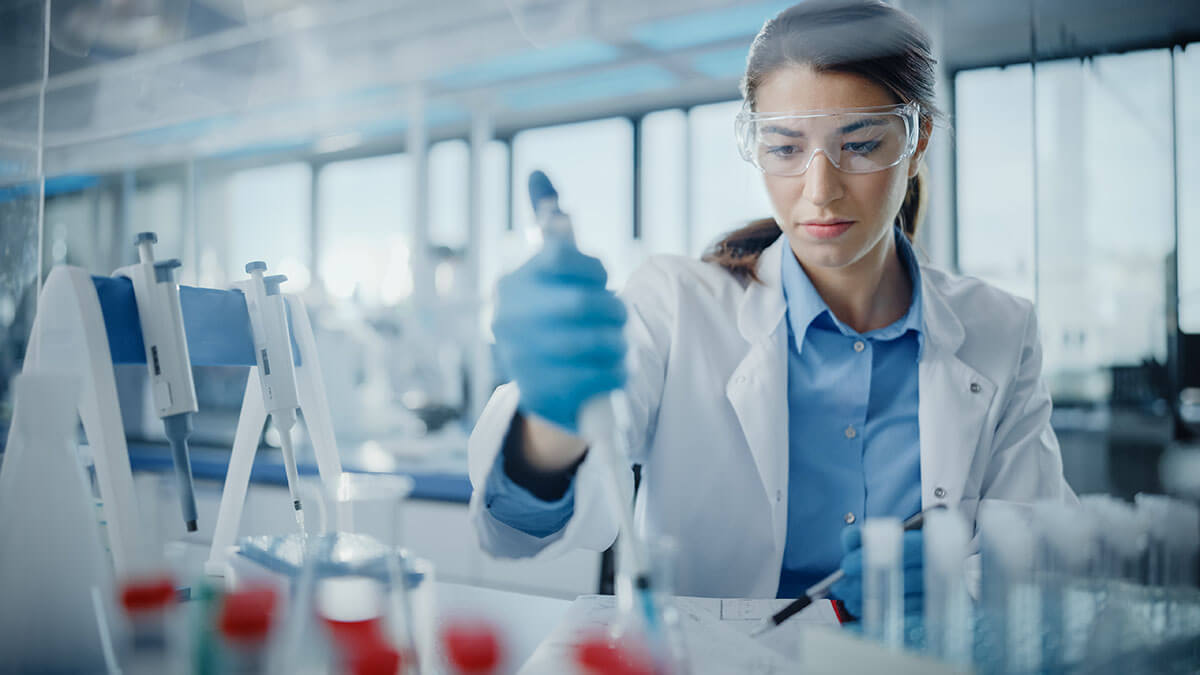
Utility of PCR blood test compared to CgA
ROC curves for the classifier applied to PCR validation set 1 and 2 compared to plasma CgA. The PCR test performs significantly (p<0.001) better.
Customers are thrilled with the results we have achieved.
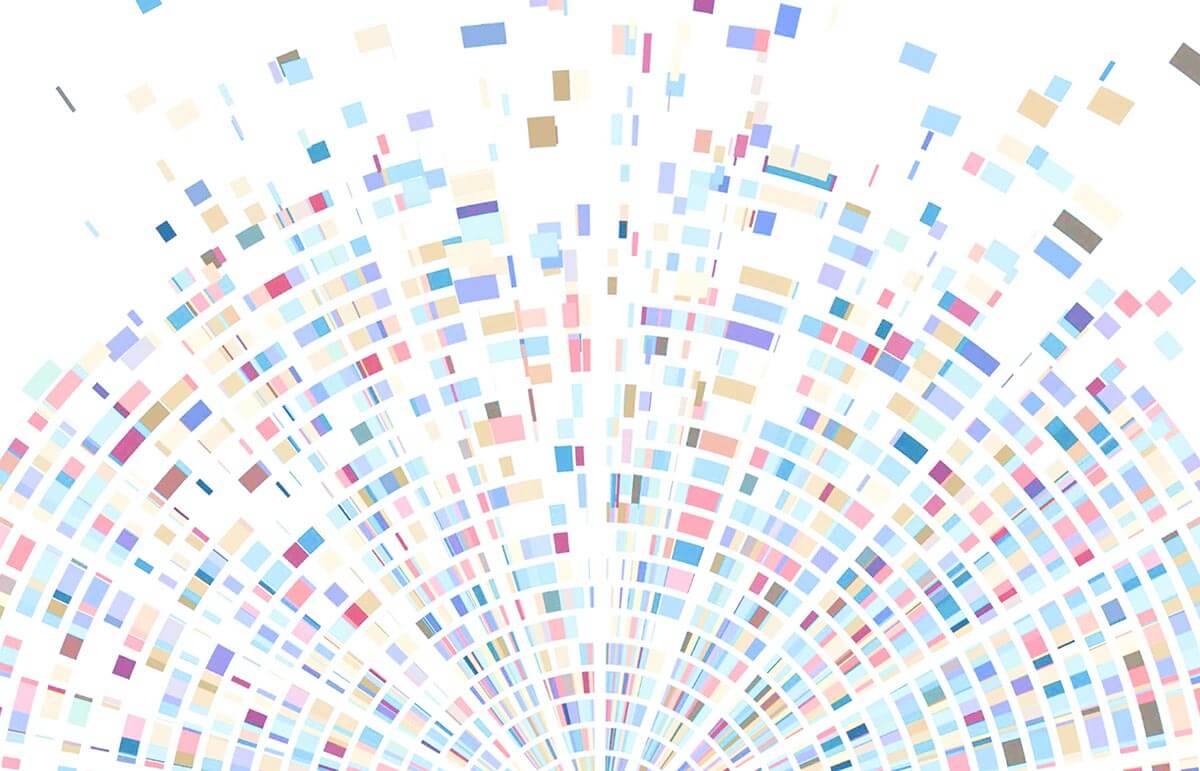